XIANG Xuezhi, a faculty member from the College of Information and Communication Engineering at HEU, recently authored a research paper published in the esteemed international journal TPAMI (IEEE Transactions on Pattern Analysis and Machine Intelligence). The paper, titled "Deep Scene Flow Learning: From 2D Images to 3D Point Clouds," delves into the realm of dense motion estimation and its applications.
Scene Flow, depicted as a dense motion field in three-dimensional space through three-dimensional displacement vectors of scene surface points in either the world coordinate system or the camera coordinate system, establishes temporal correlations within the three-dimensional structure. This allows for the accurate reflection of the actual movements of objects in 3D space. The broad applications of Scene Flow span areas such as autonomous driving, stereo video encoding/decoding, 3D scene reconstruction, human motion recognition, and advanced video surveillance.
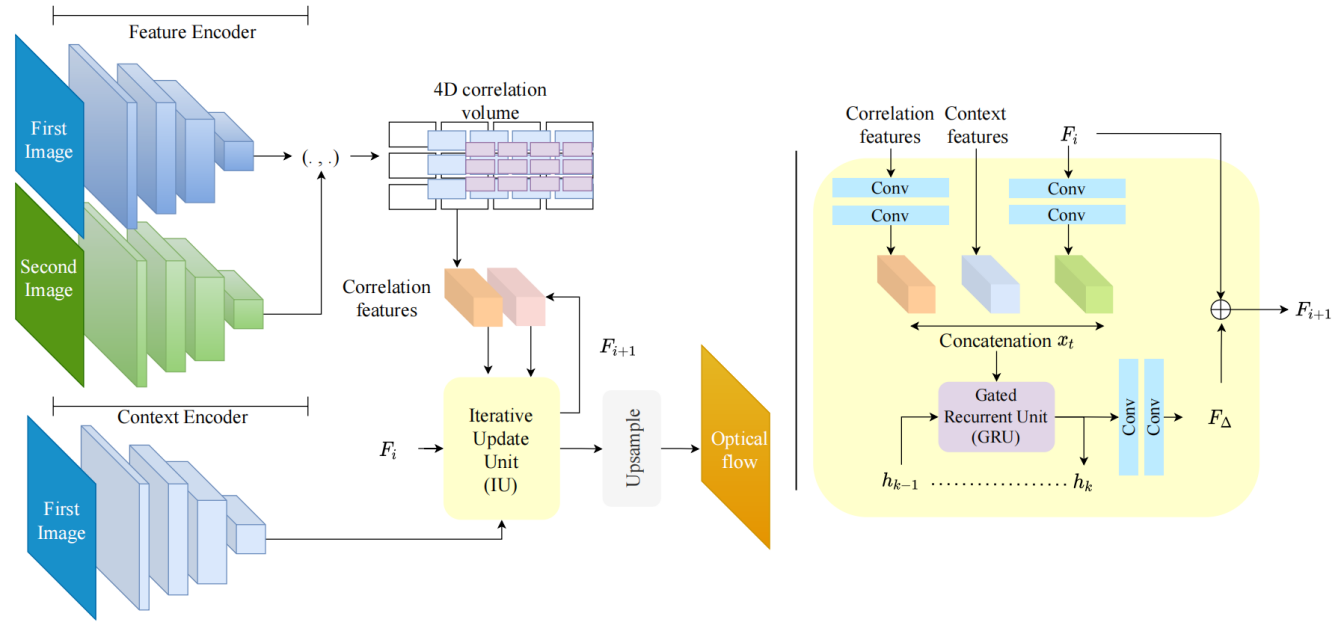
Schematic diagram of dense motion estimation
XIANG Xuezhi and his research team conducted a thorough investigation into deep learning-based scene flow estimation. They proposed a classification framework for scene flow computation, transitioning seamlessly from 2D images to 3D point clouds. The research not only explored existing challenges and model architectures but also involved a comprehensive performance and efficiency comparison among different models. The study provides valuable insights into future development trends in this field.
In their in-depth exploration, Xiang Xuezhi and his counterpart covered crucial topics related to both image-based and point-cloud-based methods. They outlined methodologies for each category, emphasizing network architecture, and conducted a detailed performance and efficiency comparison. The survey concludes with insightful discussions on open issues and directions for future research in the domain of scene flow estimation in the deep learning era.